The importance of explainable AI in a digital world
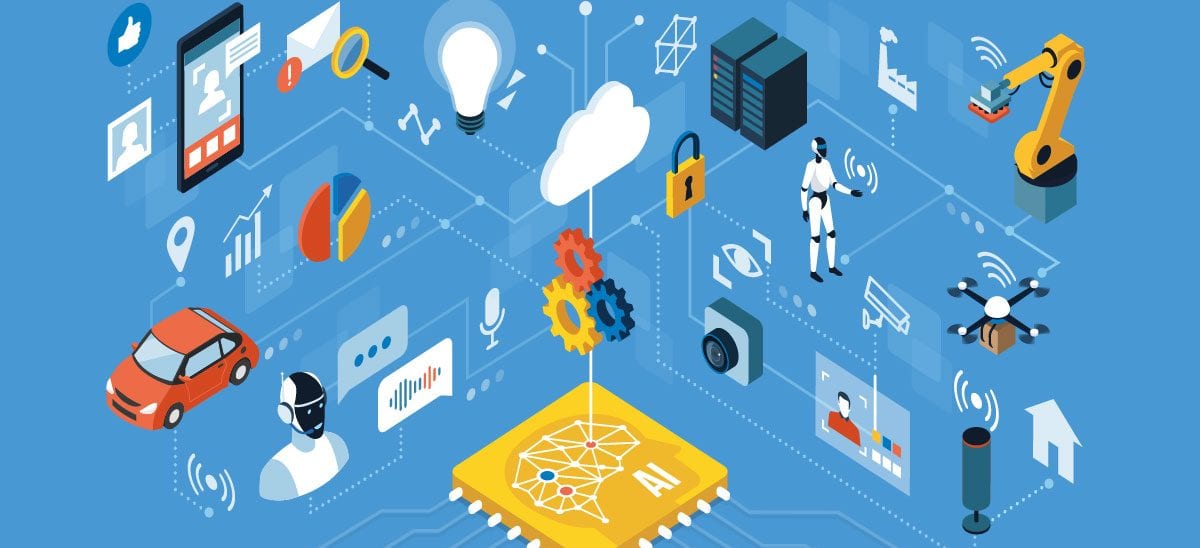
European Union (EU) lawmakers recently introduced new rules that will shape how companies use artificial intelligence (AI). At the heart of the legislation is the need to maximise the potential of AI without impeding on privacy laws or fundamental human rights. But to do this effectively, we must explain the outcomes of the AI being built – and the decisions they make. Eduardo Gonzalez, Chief Innovation Officer at Global AI Ecosystem Builder, Skymind, shares his insight.
What is Explainable AI and why does it matter?
Explainable AI is artificial intelligence where the results of the solution can be understood and explained by humans. As a problem, however, explainable AI is as old as AI itself.
Artificial intelligence is becoming a significant part of our daily lives in our digital world, from fingerprint identification and facial recognition to predictive analytics. We are finding ourselves in a position where we have no choice but to trust the outcomes of these AI-driven systems.
But how did the AI application come up with the decision it made?
You can always ask a person to explain themselves. If they decide, you can ask them why and they can then verbalise their reasoning, but that’s something that’s missing about AI.
There are a few ways that we’ve tried to make AI systems’ explainable’ – the most basic is through artificial neural networks, where algorithms are used to recognise relationships between data sets and interpret them based on what the dataset input was.
However, when it comes to things like images, machine learning can pick up on features in your dataset that you weren’t focused on and, therefore, won’t give you the desired outcomes.
For example, suppose you are trying to train the system to identify between a fox and a dog because the animal is what is very important for the AI to identify. In that case, you have to find a way to explain what the model is looking at. If you have an image of a fox and a dog and cover up the image of the fox and ask the system to identify what animal it’s looking at, and it still says the fox, then the system is not looking at the dog but something else in the image to make its decision.
The most famous case of this unexpected outcome involved when the Pentagon wanted to automatically use artificial neural networks to detect camouflaged enemy tanks automatically. They trained a neural network on photos of camouflaged tanks in trees and trees without tanks. The researchers got the results they wanted – the system worked well for them – but when the Pentagon tested it out in real life, the system failed because it was looking at the weather, not the tank. The dataset of photos of camouflaged tanks had been taken on cloudy days, while the photos of the plain forest had been taken on sunny days. The neural network had learned to tell cloudy days from sunny days instead of distinguishing camouflaged tanks from an empty forest.
AI, as it stands now, can’t do things that aren’t linear. If only we could ask the model, what is it that you’re looking at – and it could tell you, it would be easy, but it can’t communicate yet. This is why we need something explainable.
What should business leaders who are looking to embrace this technology look out for?
Leaders should first and foremost look at overcoming unconscious and conscious bias in explainable AI.
Unconscious bias is already a big problem in AI – so we need to find ways to curtail this – otherwise, businesses and countries will miss out on reaching their full economic potential.
Bias in AI can lead to all kinds of problems, such as recruiting for talent. Systems can be trained to stream resumes now, and if there’s any bias in the datasets, then the model will learn them and discriminate against candidates.
For example, a applicant might have a feminine-sounding name on a CV that a system is streaming. It won’t want to hire that potential candidate to be an engineer because of some implicit human bias against that name, and the model has picked it up and discarded the CV.
When training a model, there are ways to prevent this outcome by weighing certain things, such as giving the system a terrible score if it shows gender bias of any kind. The other way to negate this is by removing the kind of data leading to problems- so if you remove the name field in a CV – you don’t have to worry about the model learning that bias.
Explainable AI – call for a good system.
Many use cases demand and require explainable AI, and we will not get very far if we don’t have a good system for it. For example, anything that has to do with the legal system in the future that requires explainable AI could end up with many court cases being thrown out.
A good example is self-driving cars: if an accident happens and a court hearing requires an explanation as to why the computer made the decision it did, it needs to be evidenced. If the researchers of the car cannot evidence it, then the case will be dismissed.
Where will we need explainable AI the most?
Where we need explainable AI the most is in the medical field. One of the things we are doing is developing a dental AI system. We are implementing the system from a dentist’s perspective, and the things they want to see is evidence to help them come up with explanations for certain medical actions to take.
For example, the impacted tooth metric in our system grades it according to how difficult it will be addressed. A dentist can look at the x-ray, and in a few seconds, they can see what they need to do while the system is making a case for whether the patient needs to get surgery or not. Certain rules are trained to look at – such as whether the tooth’s root touches the nerve in the jaw, which can complicate things, or whether the tooth and nerve intersect.
The dentist can see where the AI system predicted the diagnosis, and they can verify and trust the machine’s decision. It decreases the workload for the dentist and saves them time having to write the report by giving them that critical second opinion.
Using the X-ray, the AI system can also flag other potential diseases not typically diagnosed with X-rays, such as cavities. Instead of the dentist having to use a magnifying glass to spend ten minutes sweeping the image, the AI system can instantly identify things they might have missed and order another test to get a better look. AI can also predict if a difficult surgery is required, which helps the dentist choose an appropriate specialist to refer to.
READ MORE:
- Biometric authentication: the good, the bad and the ugly
- You’ve had a breach – how do you successfully roll out an emergency patch?
- How to successfully reopen your office in a post-Covid-19 world
- Common migration pitfalls and how to avoid them
Explainable AI, Diversity and the Future
AI will play a much bigger part in our lives in the near future, but we need to do more to make sure that the outcomes are beneficial to the society we aim to serve. That can only happen if we continue to develop better use cases and prioritise diversity when creating AI systems. This will help mitigate conscious and unconscious biases and deliver a better overall picture of the real-world issues we are trying to address.
For more news from Top Business Tech, don’t forget to subscribe to our daily bulletin!