Could deep learning come to an end?
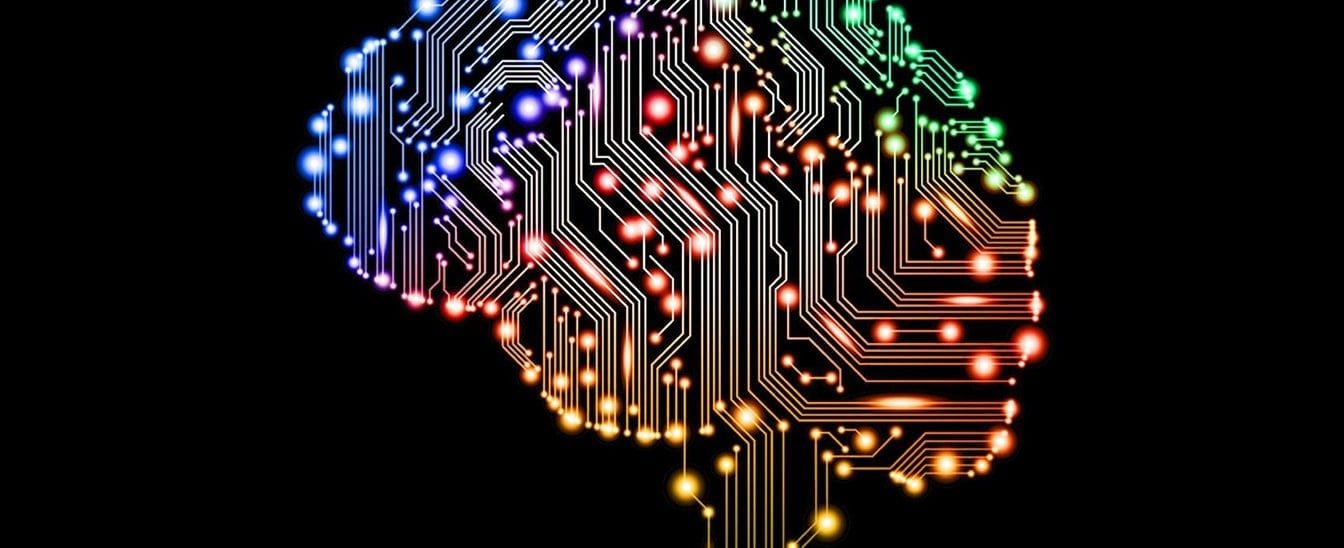
Credit: HackerNoon
Deep learning is one of the most exciting research fields in technology and the basis of so much AI: could its days really be numbered?
In 2000, Igor Aizenberg introduced deep learning in connection with artificial neural networks (ANN) to determine Boolean threshold neurons for reinforcement learning. It was a revelation. To many, it’s still the most exciting thing in artificial intelligence.
Deep learning was born in the embers of Y2K and has gone on to shape the 21st Century. Automatic translations, autonomous vehicles and customer experience are all indebted to this concept: the idea that if tech can teach itself, we as a species can simply step back and let the machines do the hard work.
Some believe that deep learning is the last true invention of the human race. Others believe it’s a matter of time before robots rise up and destroy us. We assume that AI will outlive us: what if deep learning has a lifespan, though?
MIT Technology Review looked into the history of AI, analysing 16,625 papers to chart trends and mentions of various terms to track exactly what’s risen in popularity and when. Their conclusion was intriguing: deep learning could well be coming to an end.
The emergence of the deep learning era
The terms “artificial intelligence”, “machine learning” and “deep learning” are often used as interchangeable buzzwords for any kind of computing project that requires algorithms of some kind.

This is, of course, misleading. This chart is a common visual explanation of how deep learning is merely a subsection of machine learning, and machine learning a subsection of AI.
Deep learning is but an era of artificial intelligence. MIT used the largest open-source databases of scientific papers, known as the arXiv, and tracked words mentioned to discover how AI has evolved.
These findings found three major trends. Firstly, there was a gradual shift towards machine learning that begun on the cusp of the 21st Century. Secondly, neural networks began to pick up speed around a decade later, just as the likes of Amazon and Apple were incorporating AI in their products. Reinforcement learning has been the third big wave of the last few years.
Neural networks weren’t always this popular. They peaked in the 1960s and dipped below the surface, returning briefly in the 80s and then again around 20 years later.
MIT found a transition away from knowledge-based systems (KBS) – computer programs that reason and use a knowledge base to solve complex problems – by the 21st Century. It was replaced by machine learning, which comes up with a model just from the available training data and uses that model to infer conclusions from new observations, as opposed to a KBS’s method of arriving at a conclusion based on the facts or knowledge and the “if-then” rules it has been fed.
What comes next?
There is more than one way to train a machine.
Supervised learning is the most popular form of machine learning. Decisions made in this method don’t affect what an AI sees in the future. This is the principle of image recognition: all you need is the knowledge of what a cat looks like, to recognise a cat.
Reinforcement learning mimics how we learn though: it is a sequential way of learning, meaning that that the next input of the AI depends on a decision made with the current input. Think of it more like a board game: you can play chess by learning all the rules but you truly progress as a player by earning experience.
In October 2015, DeepMind’s AlphaGo trained with reinforcement learning managed to defeat the world champion in the ancient game of Go by learning from experience. This had a huge impact on reinforcement learning. Since then, it has been picking up traction, just as deep learning experienced its boom after Geoffrey Hinton made image recognition breakthroughs towards the end of the 2000s.
AI has genre shifts like music. Just as synth-pop dominated the 80s, replaced by the grunge and Britpop of the 90s, artificial intelligence experiences the same waves of popularity. The 1980s saw knowledge-based systems dominate, replaced by Bayesian networks the following decade; support vector machines were in favour in the 2000s, with neural networks becoming more popular this decade.
Neural networks weren’t always this popular. They peaked in the 1960s and dipped below the surface, returning briefly in the 80s and then again around 20 years later. There’s no reason that the 2020s won’t bring about new changes to the way that we use AI. There are competing ideas so far about the next revolution to take hold; whatever it is could see deep learning leave the spotlight for a while.