Waymo are improving autonomous vehicles with DeepMind
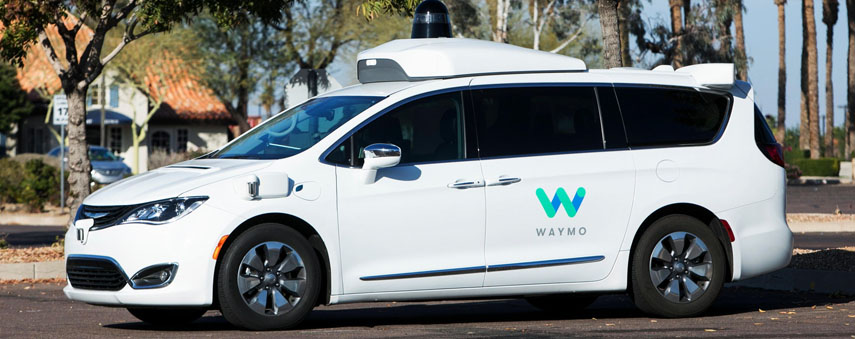
Credit: Waymo
Alphabet Inc.’s autonomous vehicle company Waymo are working with DeepMind to develop a new way to train AI, inspired by the way that biology evolves
Over 120 species are named after Charles Darwin. The effect that the naturalist, geologist and biologist had on science and nature is perhaps unparalleled in the history of humankind. Even today, his considerable influence continues to inspire the technology world.
In a research collaboration with DeepMind, Waymo has shared with the world that the two organisations are learning from Darwinian theories to make their AI training more efficient. By eliminating the weakest aspects of their training, Waymo’s self-driving algorithms essentially work on a “survival of the fittest” formula.
DeepMind claims that false positives have decreased by 24% by using Population-Based Training (PBT). This has worked out at a 50% saving of both training time and resources compared what the standard methods that Waymo previously adopted.
This could be a breakthrough in driverless technology. The sensors that are used within an automated vehicle are more than ready for public consumption, it’s just the intelligence that isn’t quite at the level required for autonomous vehicles to truly drive themselves.
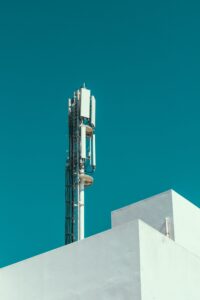
Will Waymo and DeepMind’s recent collaboration turn the key in the ignition for autonomous technology everywhere?
What is PBT?
Population-Based Training (PBT) was developed by Deepmind to find more efficient ways for training neural nets.
PBT launches lots of hyperparameter searches at once. Hyperparameters are simply parameters with values that are used to control learning; PBT compares and competes models against each other with losing models being removed from the training pool.

In the metaphor of evolution, this is a phenomenon of nature. Animals will compete for food or shelter, for example, with only the strongest, cleverest and ultimately most experienced members of a species left to reproduce. The pattern continues with the next generation. PBT is extremely similar, as “slightly mutated” hyperparameters are left standing. Hyperparameters are actively updated throughout the training.
PBT is an efficient method of machine learning as it only trains with successful hyperparameter values.
What did Waymo and DeepMind’s experiments look like?
DeepMind and Waymo first trained a network that that generates a box around road obstacles. This is called a “region proposal network”.
The goal was to see whether a PBT could improve a neural net’s capacity to identify pedestrians and cyclists, by eliminating the weaker models in the set. The PBT planned to find obstacles by recall and precision: essentially, by identifying and eliminating false positives.
“The goal of this experiment was to train this single neural net to maintain recall over 99%, while reducing false positives using population-based training,” Waymo’s blog post read.
In these experiments, we were able to increase diversity by creating sub-populations called “niches,” where neural nets were only allowed to compete within their own sub-groups–similar to how species evolve when isolated on islands. We also tried to directly reward diversity through a technique called “fitness sharing,” where we measure the difference between members of the population and give more unique neural nets an edge in the competition. Greater diversity allows PBT to explore a larger hyperparameter space.
Waymo
The experiment had some interesting results. Waymo found that they needed to evaluate networks to find if they would perform to their capacity in the real world; after all, neural nets need to be able to react to their surroundings, rather than simply pull up learned information.
Waymo separated PBT competition sets from training sets, before verifying final performance with an evaluation set, that the neural nets have never seen in training or competition. Think of it as training for your driving test: rather than just reading the highway code, your experience comes from the practice you’ve had in driving lessons and other driving tests should you have failed before. This forms your final performance in the same way as these competition and training sets.
Waymo also relied on Google’s data centres. The PBT models used were evaluated every 15 minutes in order to weed out the weaknesses across hundreds of machines.
What did Waymo discover?
PBT was found to reduce a lot of time and effort, as models were constantly competing against each other. Not only that, but PBT uses half the computational resources used by random parallel search.
PBT is now being used by a host more Waymo vehicles. It’s hoped that this efficiency and evolutionary tactic will help to build better, more efficient driverless cars even quicker.
Evolution continues to inspire. Who knows where the world of autonomous vehicles will develop in the next few years?